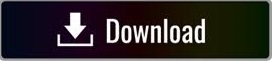
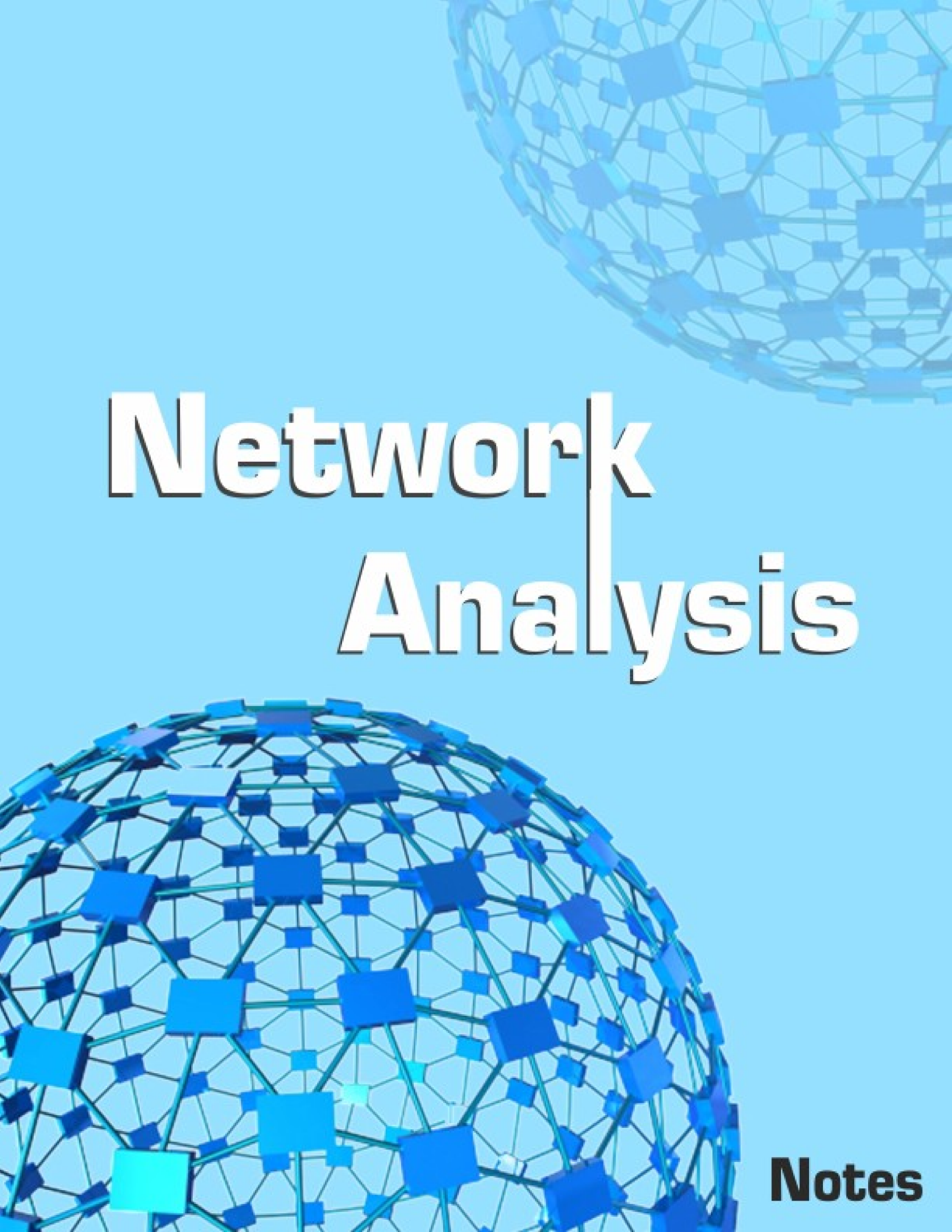
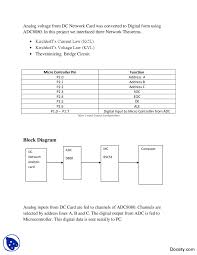
The resulting entropies have proved to be useful in classifying graphs according to structure, and the computational complexity is polynomial. For instance, Dehmer has proposed a generalized framework for defining graph entropies based on the development of the graph topological information functionals. Shannon’s entropy function can be directly applied to a probability distribution whose values are assigned by functions that capture the structural characteristics of a graph.

These shortcomings seriously limit the direct use of Körner’s entropy in the field of network analysis.Ī number of alternative methods therefore use Shannon’s entropy for quantifying the complexity of a graph. Another drawback of this approach is that it is not applicable to more general large-scale graphs. Unfortunately, as this complexity measure is posed as a coding optimization problem in information theory, it cannot be used as a quantity to reflect the graph structural properties. Extending this idea to graphs, the Körner’s entropy is defined as the minimal cross entropy between the vertex packing polytope and the vertex probability distribution. The original motivation of this measure is to compute the entropy of an information source with ambiguous alphabet. One of the earliest and classical contributions in randomness complexity is Körner’s entropy associated with a graph. For instance, one approach is to use entropy to characterize the degree of randomness in a network. The problem of determining the homogeneity of network substructure can also be posed in terms of entropy, and there are a plethora of alternative ways in which network entropy can be measured. Such measures prove to be powerful when the analysis of highly inhomogeneous structures such as protein–protein interaction networks is attempted. This analysis can be extended to edges, and leads to the definition of network characteristics, such as the Estrada index which aims to measure the variance of vertex-degree across edges in a network and allows the homogeneity of networks to be assessed. Vertex degree distributions have proved to be a powerful tool in the analysis of complex networks, and allow different network behaviours, such as the Erdős–Rényi, ‘small-world’ and ‘scale-free’ models to be easily distinguished. We apply the resulting methods to the problem of graph classification, and compare the results obtained to those obtained using some alternative state-of-the-art methods on real-world data. We explore a number of variants of this method, including using both fixed and adaptive binning over edge vertex degree combinations, using both entropy weighted and raw bin-contents, and using multi-linear PCA, aimed at extracting the tensorial structure of high-dimensional data, as an alternative to classical PCA for component analysis. By performing principal component analysis (PCA) on the feature vectors for samples, we embed populations of graphs into a low-dimensional space. We normalize the resulting histograms and vectorize them to give network feature vectors reflecting the distribution of entropy across the edges of the network. Each edge in a network increments the contents of the appropriate bin in the histogram, indexed according to the degree pair in an undirected graph or the in/out-degree quadruple for a directed graph. Since our entropy is defined in terms of vertex degree values defining an edge, we can histogram the edge entropy using a multi-dimensional array for both undirected and directed networks. The aim in this paper is to use our previous analysis to define a new characterization of network structure, which captures the distribution of entropy across the edges of a network. However, this analysis was posed at the global level, and did not consider in detail how the entropy is distributed across edges. Recently, we have reported an approximate network von Neumann entropy measure, which can be conveniently expressed in terms of the degree configurations associated with the vertices that define the edges in both undirected and directed graphs. For instance, entropy can be used to distinguish between different structures. Structural complexity measures have found widespread use in network analysis.
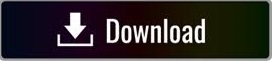